When wars occur, the side that boasts comparatively greater military power is at an advantage but does not always emerge victorious. Why? Several theories offer ostensible answers—war weariness, risk aversion, military myopia, problems with civil-military relations, miscalculations. We suspected that if we knew how states (and nonstate combatants) fight, we could better answer this consequential question. Aphorisms about winning the battle but losing the war highlight a problem with current approaches. There is a missing middle, an underappreciated meso-level of conflict: operations. Alas, there were little to no organized data at this level.
Several important and interesting questions can be and have been answered by case studies at the operational level. There is a whole host of questions, however, that require a broader scope. There are systematic patterns and lessons on how the US military fights, whether it wins or loses battles and wars, that only quantitative data can resolve. To date, this has only been available at the war level or piecemeal, leaving much to be desired where the actual warfighting occurs.
Most existing datasets dwell at the strategic level, collapsing all information on military interventions into one aggregated line item. For instance, three seminal data sources—the Military Intervention by Powerful States project, the International Crisis Behavior project, and the Militarized Interstate Disputes data set—have a single observation for the two-decade Afghanistan War. This drastically masks the prosecution of war, precluding analyses of how states fight, obscuring ebbs and flows over the course of the war, and limiting our ability to pinpoint and parse wars’ causes and effects.
Some studies address this by analyzing specific technologies and innovations, and we have certainly gained much from focused analyses of particular military means like nuclear weapons, naval power projection, aerial bombing, ballistic missiles, cruise missiles, or drones. But this work involves several limitations. First, it tends to focus on the possession of these platforms rather than their use, offering insight only on the latent potential for force. Second, most focus narrowly on a single platform. This overlooks that leaders craft the use of military force in packages and from a menu of substitutable (or complementary) options. Furthermore, while tracing more precise stages of wars it primarily details those where a platform of interest is in play. Like visual closure, this forces scholars to assume or imagine the complete picture of a war with incomplete information.
Finally, the datasets capturing cases within wars do not record the relations between them. Returning to the Afghanistan War, RAND improves on sources listing one line item by including more detailed information on more specific subcomponents like Operation Enduring Freedom, Operation Swift Freedom, the International Security Assistance Force, Resolute Support, and Operation Freedom’s Sentinel. However, not only does this blur levels of aggregation—since some of these are campaigns, some are subcampaigns, and some are operations—but it also fails to depict that lower-order cases are nested within broader ones. Figure 1 illustrates the unstructured list alongside the structured reality. Rather than independent observations arising in isolation, Operation Swift Freedom was part of Operation Enduring Freedom–Afghanistan, Operation Freedom’s Sentinel was the US component of NATO’s Resolute Support mission, and of course all of these occurred under the umbrella of the Afghanistan War.
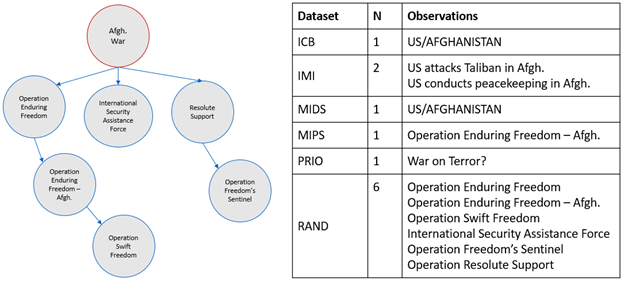
Overall, existing resources are either highly generalized on one side or highly specific and siloed on the other and none address how observations relate to one another.
MONSTr in the Middle
We created Military Operations with Novel Strategic Technologies in r (MONSTr), an open-source and publicly available dataset and website that features:
- measures of the means of military force (the how) across
- a comprehensive and disaggregated list of US military operations from 1989 to January 2021 (neither generalized nor siloed) that
- captures dependence between observations.
Interested in what happens in military interventions at the operational level, we followed the path cleared by the Military Intervention by Powerful States and International Military Intervention datasets to identify interventions and the Department of Defense Dictionary and Joint Publication 3-0 to scope to the operational level within them. For the dataset, we defined a military operation as:
a series of tactical actions (battles, engagements, strikes) conducted by combat forces in an operational theater to achieve strategic or campaign objectives in the context of a political issue or dispute through action against a foreign adversary.
Data collection is a titanic, labor-intensive, meticulous task. The only way we were able to accomplish what past efforts could not was to embrace state-of-the-art automated data retrieval techniques from a taboo source: Wikipedia. We scraped the structured knowledge graphs of this familiar, yet underutilized source using SPARQL, a customizable database query language. For any sticklers, techies, or skeptics, we discuss our definition work, Wikipedia’s anatomy, details of the extraction process, audits, and validation exercises in our recently published debut article.
Features and Uses
MONSTr’s unit of analysis is more granular, its depth more detailed, and its structure more realistic and scalable than existing data sources. First, by disaggregating the unit of analysis from wars to individual operations, we provide information on 313 observations representing the sixty-five post-1989 US interventions covered in all existing datasets combined. We include variables identifying the strategic events in which our cases are nested so that scholars can isolate them, cross-reference with other sources, and scale up and down at will.
Second, we extracted several covariates characterizing military operations. For instance, we indicate the means of force employed in each event. Other datasets either code domains (i.e., air, land, or sea) or exclusively list a sole type of force. We identify seven types: ground troops, paramilitary (special operations), close air support, air-to-air combat, aerial bombing, cruise missiles, and drones. The categories were informed by several things. First, this is largely how scholars have broken out the means of force in studies examining them in isolation. Second, the military and government generally think about force employment this way—cruise missiles were selected as the means of achieving the intended objectives in Operation Infinite Reach, for example, while the use of drones and numbers of ground troops provided frames of reference in Afghanistan. Third, it coincides with the way that Wikipedia distinguishes actions, differentiating between drones and piloted aircraft but seldom between tanks and transport vehicles.
Each military approach has its pros, cons, and fitness for a given operational environment. Additionally, each sends a different signal of risk tolerance or resolve. Of course, force planning and employment also depend on the technologies available. Figure 2 shows a cumulative count of each type of force we track. We began with 1989, marking the advent of consistent US cruise missile use in conflict, yet this platform has not been used in public view as extensively as some predicted. On the other hand, the use of special operations forces intuitively increased to intermix advanced combat technology and population trust in the counterinsurgency campaigns in the Afghanistan and Iraq Wars.
Our dataset also enables analyses of combined force employment showing which means were used in conjunction. As modern warfare becomes more complex and multidomain, this information is critical to empirically examine how the US military employs jointness in action.
MONSTr provides a replete list of combatants on both sides (allies and adversaries) for every operation. The identity, traits, and dynamics of conflict actors strongly influence how they fight. Many data sources, however, generalize this aspect by looking at the primary intervener and target. This neglects vital and nuanced variation in war participation, especially ones occurring over years or even decades. The rosters comprising the coalition and the targets throughout the Afghanistan War, for instance, shifted as it unfolded.
Simply look at the top right panel of Figure 3, plotting only US allies, to get a sense of this diversity and dynamism. Compared to partners in the 1991 Gulf War (all of which were states) and the later Syria War (most of which were nonstate combatants), we see temporal trends and idiosyncrasies ripe for exploration. Especially as conflicts increasingly feature nonstate actors, we must unpack how the United States fights alongside or against them given asymmetry in strength and unconventional strategies. The dataset supports unprecedented assessments of longevity, loyalty, alliances of convenience, side switching, and more.
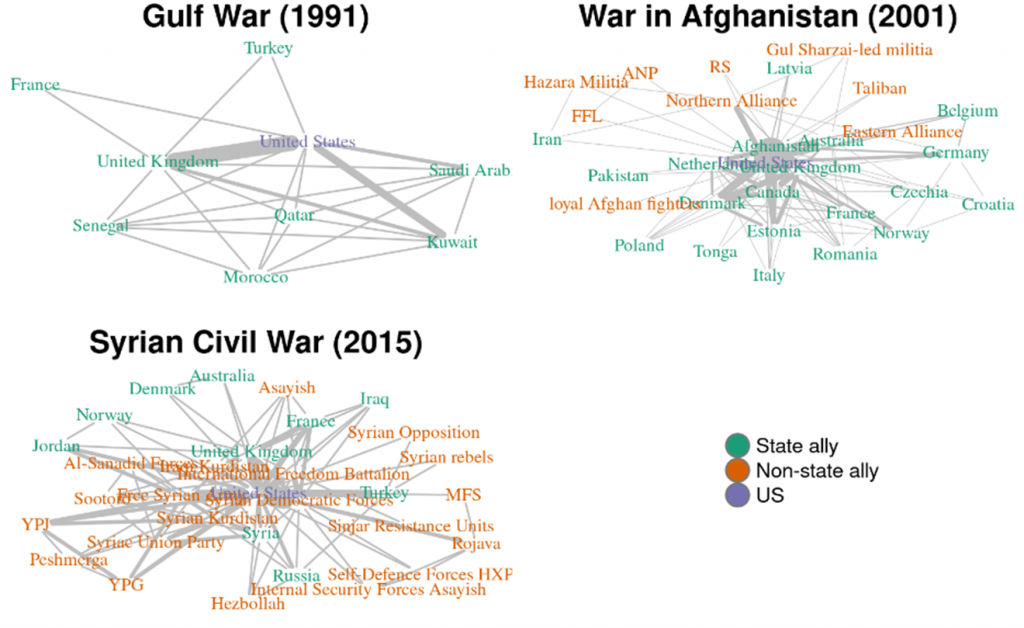
MONSTr explodes the number and direction of avenues for future research on the geography and temporality of war. Nearly all operations are geocoded with GPS coordinates or the name of a local municipality. Spatial analysts can investigate how borders, terrain, degree of urbanization, resource access, strategic sites, infrastructure, and many more variables affect the application of military force.
Perhaps more pioneering, the dataset’s nested structure identifying operations within broader interventions will equip researchers to study time and context in war with greater rigor. For example, we code how many days into the parent war (or campaign) each operation occurred. This spotlights several questions. For instance, how do wars change across their life cycles, in real time, in response to withdrawals and insertions, operational failures and successes, casualties (deeply studied yet still disputed and conditional), public opinion shifts, elections and other domestic political developments, leader turnover, and even seasons? This also enables researchers to reverse the causal arrow to explore a new set of questions where the tides of war are the cause rather than the outcome.
Our data also enable more expansive inquiries of legacy theories. By controlling for the strategic context in which operations occur, it is possible that researchers have over- or underestimated the impact of certain determinants on force employment, especially structural factors. As an example, Figure 4 shows the means of force used in operations within the three primary campaigns of the Gulf War: the Iraqi no-fly zones, the air campaign, and the liberation of Kuwait. It is no surprise that the air campaign featured aerial sorties and bombing while the liberation campaign primarily comprised ground troops with close air support. By accounting for the strategic objective defining a cluster of operations, scholars can more accurately model the causes of force employment choices and the effects of their performances.
MONSTr exists here as both a static dataset and interactive website. We hope it grows. More can, should, and will be done to identify and explain military operations across more expansive time periods, more states, and more detail. We invite practitioners, students, and quantitative scholars of war to browse and use it.
Kerry Chávez, PhD, is an instructor in the Political Science Department and project administrator at the Peace, War, and Social Conflict Laboratory at Texas Tech University. She is also a nonresident research fellow with the Modern War Institute at West Point. Her research focusing on the politics, strategies, and technologies of conflict and security has been published in several venues including the Journal of Conflict Resolution, Foreign Policy Analysis, War on the Rocks, and others. With practitioner and law enforcement experience as well as working group collaborations, she produces rigorous, engaged scholarship.
J. Andrés Gannon, PhD, is an assistant professor of political science at Vanderbilt University and a faculty affiliate at the Data Science Institute. Previously, he was a Stanton nuclear security fellow at the Council on Foreign Relations, a nonresident fellow at the Eurasia Group Foundation, an International Security Program postdoctoral research fellow at the Harvard Kennedy School’s Belfer Center for Science and International Affairs, a Hans J. Morgenthau research fellow at the Notre Dame International Security Center, and a PhD Eisenhower defense fellow at the NATO Defense College.
The views expressed are those of the authors and do not reflect the official position of the United States Military Academy, Department of the Army, or Department of Defense.
Image credit: Staff Sgt. Alex Ramos, US Army